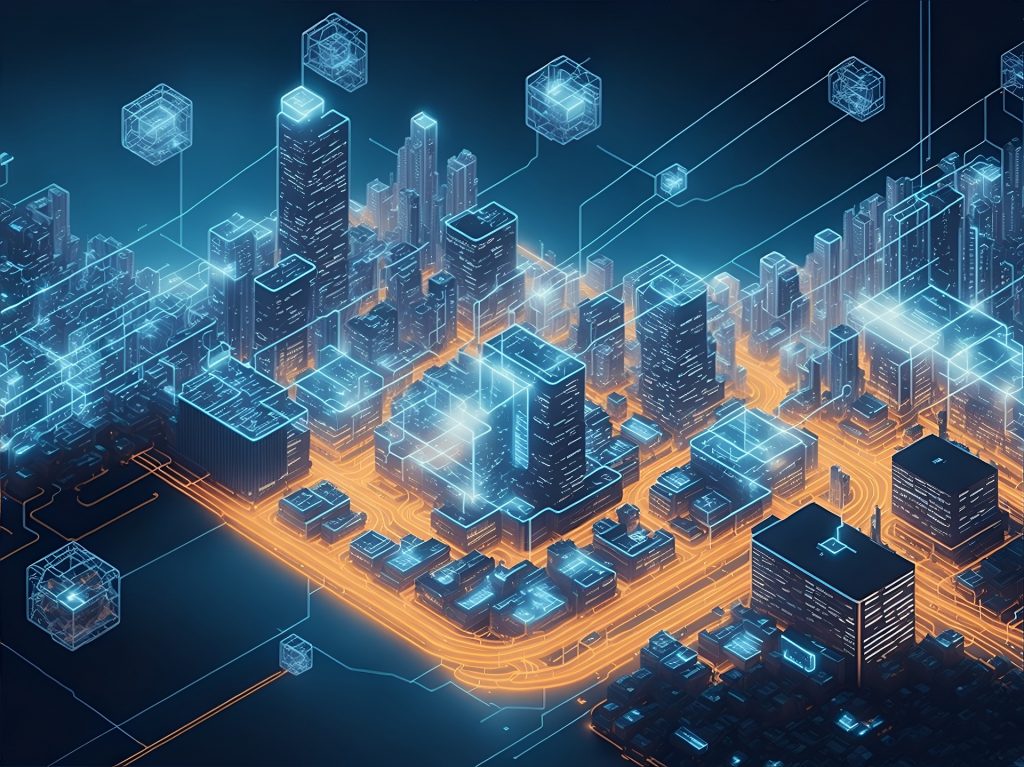
The axis of Artificial Intelligence (AI) training has traditionally spun around the acquisition and storage of colossal volumes of real-world data. This conventional approach, while effective, poses several challenges including storage costs, data privacy concerns, and the ecological footprint of data centers. However, the innovation of synthetic training data is ushering in a compelling alternative. Models such as Wayve’s GAIA-1 and platforms like the Universal Simulator (UniSim) are at the forefront of this transition, showcasing the ability to generate synthetic data for training AI models, potentially reducing the reliance on stored real-world data.
GAIA-1: A Forerunner in Synthetic Data Generation
Unveiled in June 2023, GAIA-1 emerged as a groundbreaking generative model aimed at enhancing the resolution of generated videos and improving the world model quality through larger-scale training. This 9-billion parameter generative world model is designed to offer a structured understanding of environmental dynamics, crucial for making informed decisions while driving. The model’s adeptness in accurately predicting future events is seen as a cornerstone for enhancing safety and efficiency on the roads, allowing autonomous vehicles to better anticipate and plan their actions in real-world scenarios.
UniSim: Bridging the Synthetic Data Gap
On another spectrum, the Universal Simulator (UniSim) project explores the potential of synthetic data in simulating realistic interactions between humans, robots, and other interactive agents. By emulating human and agent interactions with the world, UniSim provides a glimpse into a future where AI systems can be trained using generated data, eliminating the need for storing extensive real-world datasets. The simulator has shown promising results in training both high-level vision-language planners and low-level reinforcement learning policies, exhibiting significant transfer from training in a simulator to real-world scenarios.
The Speed of Data Generation Versus Ingestion
The remarkable pace at which synthetic data can be generated presents a nuanced challenge—the disparity between the rate of data generation and the rate of data ingestion for training purposes. The rapid generation of synthetic data may outpace the ability of AI models to process it in real-time. This scenario underscores the possible necessity for a caching mechanism to temporarily store generated data, ensuring a continuous and efficient training pipeline. While this doesn’t equate to the long-term storage of real-world data, it hints at a nuanced approach where temporary storage of generated data bridges the gap between generation and ingestion.
The Transition to Caching Generated Data
This rapid generation of synthetic data, although a boon, necessitates a strategy to address the lag in data ingestion rates. Caching emerges as a viable solution, acting as a conduit between data generation and data ingestion, ensuring a seamless training process. This approach, while not entirely eliminating the need for data storage, significantly reduces the volume of data that needs to be stored and managed over time.
Conclusion
The advancements in synthetic data generation as demonstrated by GAIA-1 and UniSim are redefining the landscape of AI training. The age-old practice of storing vast amounts of real-world data for training purposes might soon be eclipsed by more efficient, and scalable, synthetic data generation methodologies. The unfolding narrative in this domain is not only promising but indicative of a future where the training of AI systems is constrained only by the bounds of creativity, not storage capacity. The narrative around synthetic data resonates with the axiom—architecture matters. The pivot towards synthetic data underscores a significant architectural shift in AI training, which is bound to have a ripple effect across the broader spectrum of AI and machine learning domains.
- Universal Simulator (UniSim): universal-simulator.github.io/unisim
- Scaling GAIA-1: wayve.ai/thinking/scaling-gaia-1